SelfAutoDOC - Self-healing Automotive Driving platform powered by Organic Computing
Project Description
Future autonomous vehicles with complex functions must be able to react flexibly to various error influences. This flexibility and adaptability are currently guaranteed by the human driver. With classic approaches, errors and associated countermeasures are statically modeled. However, the complexity and variability of possible error scenarios is so high that it can no longer be efficiently and comprehensively modeled using static techniques. Rather, what is needed is a system that adapts dynamically to an emerging scenario and initiates appropriate countermeasures. In addition, the system should proactively adapt to changes in order to counteract the occurrence of errors at an early stage. It is precisely these properties that are to be addressed in the SelfAutoDOC project: Organic Computing transfers self-sustaining concepts from biology to information technology. The systems developed with these concepts have, among other things, the property of being able to configure themselves, adapt and, in particular, be able to heal themselves. In connection with techniques of active error diagnosis, current causes of errors and future error potentials are identified in a fine-grained manner, so that the reliability and availability of the system services are significantly increased by means of targeted interaction. In this project, such organic computing techniques are to be developed and evaluated using real vehicle hardware (hardware in the loop, possibly a test vehicle) and for vehicle functions. For this purpose, the steering or braking function is implemented with the help of organic computing methods and the correct behavior is evaluated in driving tests. The main innovation of the project is the research and testing of the use of organic computing techniques in distributed vehicle systems. In particular, the combination with time-triggered automotive networks as well as organic computing diagnosis and its integration with self-healing will be investigated. This is intended to achieve increased reliability, which allows safety-critical fail-operational automotive systems with lower costs and greater flexibility compared to active redundancy. At the same time, the formal verification, and the further optimization through connection to the system diagnosis will be researched.
SelfAutoDOC is funded by the Federal Ministry for Economic Affairs and Energy based on a resolution by the German Bundestag.
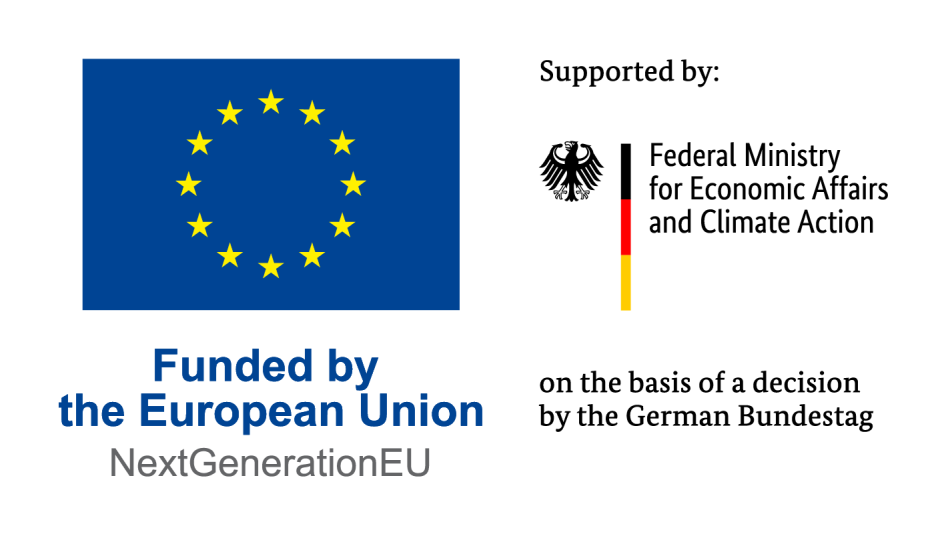
One of the main goals of TTTech Auto Germany in SelfAutoDOC is to expand the middleware software with reallocation services and reconfigurations that are required for porting AHS (Artificial Hormone System and ADNA (Artificial DNA). In addition, TTTech Auto Germany will develop self-healing concepts based on middleware and time-triggered communication services using ADNA and AHS. Furthermore, we will carry out the formal verification for the algorithms developed in the middleware. In addition, TTTech Auto Germany examines and identifies the status variables relevant for diagnosis in the middleware and also contributes to the implementation and demonstration of the project results, as well as the experimental and analytical evaluation.